Ploting in Pandas#
Having to load data manually to build a visualization or plot gets cumbersome quickly. In many situations, we might want to work with data in a pandas
DataFrame
when building a visualization.
The pandas
.plot()
attribute relies on the matplotlib
API to generate plots, so our work with matplotlib
will come in handy when we need to customize plots generated using .plot()
. And in many cases, the .plot()
syntax is similar to matplotlib
OO
syntax.
We’ll start by working with air quality data.
.plot()
#
We can do a quick visual check of the data by passing the entire DataFrame
to .plot()
.
import pandas as pd # import statements
df = pd.read_csv('https://raw.githubusercontent.com/kwaldenphd/elements-of-computing/main/book/data/ch10/air_quality_no2.csv', index_col=0, parse_dates=True) # load data
df # show output
station_antwerp | station_paris | station_london | |
---|---|---|---|
datetime | |||
2019-05-07 02:00:00 | NaN | NaN | 23.0 |
2019-05-07 03:00:00 | 50.5 | 25.0 | 19.0 |
2019-05-07 04:00:00 | 45.0 | 27.7 | 19.0 |
2019-05-07 05:00:00 | NaN | 50.4 | 16.0 |
2019-05-07 06:00:00 | NaN | 61.9 | NaN |
... | ... | ... | ... |
2019-06-20 22:00:00 | NaN | 21.4 | NaN |
2019-06-20 23:00:00 | NaN | 24.9 | NaN |
2019-06-21 00:00:00 | NaN | 26.5 | NaN |
2019-06-21 01:00:00 | NaN | 21.8 | NaN |
2019-06-21 02:00:00 | NaN | 20.0 | NaN |
1035 rows × 3 columns
df.plot() # plot entire dataframe
<Axes: xlabel='datetime'>
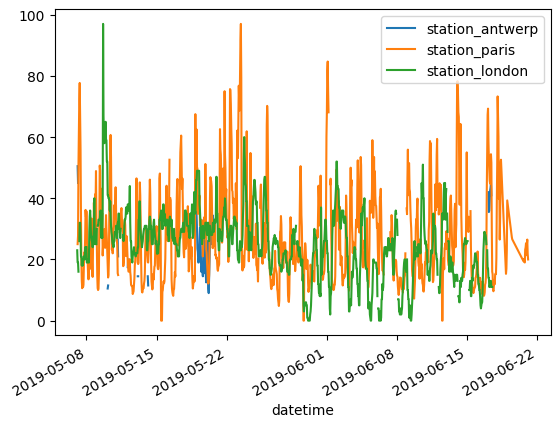
This isn’t a particularly meaningful visualization, but it shows us how the default for .plot()
creates a line for each column with numeric data.
Default settings for Pandas
plotting functions:
index
(in this caseDateTime
) is thex
axis dataNumeric columns are the
y
axis dataDefault plot type is a line plot
Default axis title(s) and legend are pulled from information in the underlying
DataFrame
Plotting Specific Columns#
Let’s say we only wanted to plot Paris data. We can plot a specific column in the dataframe
using the [" "]
selection method before calling .plot()
.
df["station_paris"].plot() # plot specific column
<Axes: xlabel='datetime'>
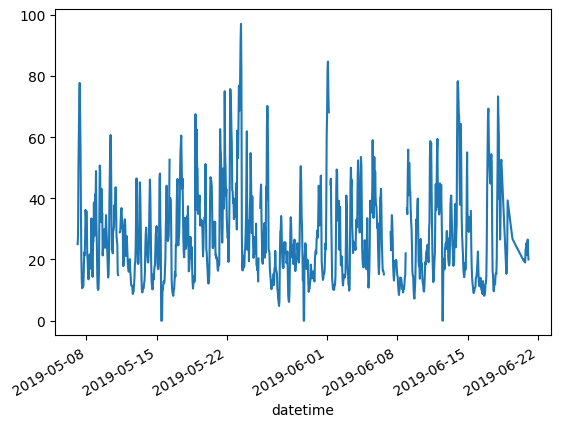
Let’s say we want to visually compare NO2 values measured in London and Paris. We need to specify what column is going to be used for the X
axis as well as what column is going to be used for the Y
axis.
For this example, a scatterplot will be more effective than a lineplot, so we’ll use .plot.scatter()
.
df.plot.scatter(x="station_london", y="station_paris", alpha=0.5) # scatterplot for two columns
<Axes: xlabel='station_london', ylabel='station_paris'>
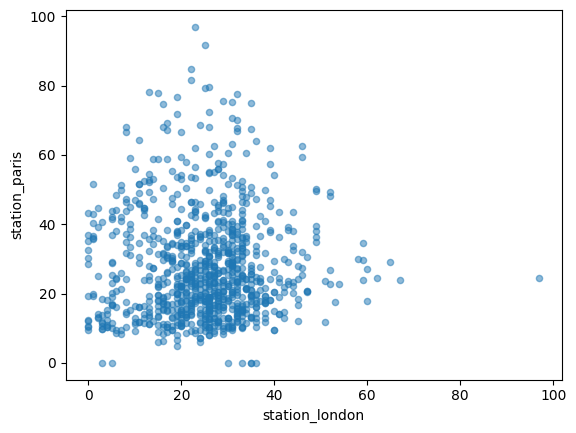
Additional Line Plot Resources#
For more on line plots:
Additional Scatter Plot Resources#
For more on scatter plots:
Recap#
As we’ve already seen Pandas
plotting functions incorporate a mix of Pandas
syntax and arguments that share similarity with matplotlib
.
We’ll see that pattern continue as we explore other plot types and plotting workflows.